Recommendation systems: your personalized preferences or just what’s popular?
Companies like Google, Apple, Facebook, Amazon, Spotify and Netflix use algorithms to try to offer their users personalized recommendations. Two Spanish researchers found that even in these personalized suggestions, the views of the majority carry a heavy weight.
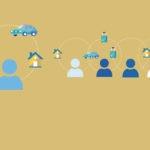
Recommendation system algorithms are an essential mechanism in digital consumer markets. When they’re right, they get users to spend more time on platforms like YouTube, Amazon, Netflix and Spotify, opening the door to more advertising dollars or direct sales. But how exactly do they work?
In theory, the success of these algorithms depends largely on personal preferences. Everyone is different and that means that the algorithm must be able to learn from each user’s preferences in order to offer what they really like. But the fact that a song, movie or video has been wildly successful also plays a big role. To what extent does popularity prevail over personal preferences in the algorithms?
Pablo Castells and Rocío Cañamares are two computer engineers from the Autonomous University of Madrid, who tried to answer this question in their study, “Should I follow the crowd? A probabilistic analysis of the effectiveness of popularity in recommender systems”. Their work was recognized as the best paper at the 2018 international ACM SIGIR Conference on Research and Development in Information Retrieval, heldin Ann Arbor, Michigan in July.
In theory, customization is what gives users the greatest satisfaction, but to what extent is it essential to keep pushing in this direction? “If popularity works, why get rid of it?” wonders Castells, Associate Professor at the Superior Polytechnical School at the Autonomous University of Madrid. Clearly the recommendation system looks for what’s new to help users discover something they don’t already know, and what’s popular with most people is very new by definition. In other words, an algorithm that recommends listening to Madonna and visiting the Eiffel Tower in Paris would be pretty useless.
"Pioneering work is already underway in the financial sector, where the concept of “fairness” is explicitly codified in artificial intelligence"
At the same time, “researchers have realized that the recommendations that the supposedly personalized algorithms make tend to be what the majority prefers,” explains Castells.
“There is a bias toward what’s popular in the personalized algorithms that apparently work best,” says the professor. His acclaimed research concludes that most recommenders get it right when the algorithm also accounts for the user’s past preferences.
Furthermore, the algorithm is spot on when that “vote of the masses” is the only factor in the formula. Mistakes and distortions appear when the algorithm integrates other factors, such as an advertising campaign in addition to popularity and the user’s previous preferences.
There is no definite answer, but talking to Castells and Cañamares in a quiet office at the Autonomous University of Madrid, it is clear that personal preferences are probably less decisive than users would like to believe. “I’m not an anthropologist,” says Castells, “but we can’t forget that majorities are based on imitation, and imitation is a vital mechanism for the human species. You spend the early years of your life imitating what you see around you. Thanks to this, you absorb thousands of years of civilization, you learn a language, and without a doubt, you survive.”
The power and responsibility of an algorithm
“Fake news” during the 2016 U.S. presidential elections put Facebook and its algorithm in the spotlight and reignited debate about the power of algorithms. Their “artisans” - engineers and computer scientists - create formulas that aim to meet a corporate target: more clicks, more users, more time on platforms.
The problem is that these premises can have very dangerous consequences. If Facebook’s algorithm sees clicks on a “news story” - regardless of how ridiculous it may be (like Pizzagate, the fake story about a network of pedophiles tied to Hillary Clinton based out of a Washington DC pizza shop) - it offers more content of a similar nature, thus creating a bubble of “fake news”. Similarly, YouTube tends to offer more and more extreme, radical content to users who watch violent videos.
Is the algorithm perverse? Quite the contrary, says Cañamares: “It’s completely innocent. It sees that you like something and offers you more of the same, without giving it a second thought. That’s where engineer comes in - to add nuances.”
“The metrics that interest business executives the most are dollars and sales,” adds Castells, “but there is increasingly more awareness of the need to add a ‘fairness’ criterion.” In other words, eliminate racial, radical or sexist biases from the algorithm’s recommendations, or try to encourage a range of different views for controversial topics.
In fact, pioneering work is already underway in the financial sector, where the concept of “fairness” is explicitly codified in artificial intelligence, making it measurable and auditable. BBVA Data & Analytics’ study “Reinforcement Learning for Fair Dynamic Pricing” is one example. In this article, the BBVA data scientists explain how to conduct dynamic pricing using an artificial intelligence model that includes fairness principles to avoid discrimination. In their study, the researchers propose a metric designed to analyzed “how fair” dynamic price policies are and integrate these measures in the optimization process. Therefore, thanks to their work, it’s possible to constantly monitor and audit the algorithm’s fairness.
Therefore, the algorithms are like a potion with lots of ingredients: what’s popular, mixed with personalized preferences and new considerations, and a splash of the “fairness” criteria to avoid distortions.
Castells compares creating a recommendation system algorithm to building a prototype of a car with thousands of screws that are tightened little by little, even under real conditions. “The tech company that prevails in the end will be the one with the best engineers behind their algorithms and at the same time, the one that is able to spend more time supervising, adjusting and correcting,” he predicts.
BBVA Data & Analytics recently prepared a piece to explain how the different recommendation systems work in the best known digital platforms, and how they could be expanded to other industries. The result was RecSys, a recommendation in the age of machine learning. It explores the possibility of algorithms finding “unexpected connections” in the data to offer users recommendations based on non-linear relationships that can more precisely capture the way people relate concepts.