BBVA teams up with MIT to improve card fraud detection
BBVA collaborated with a research team from the Massachusetts Institute of Technology (MIT) to develop a model that can reduce the level of false positives in fraudulent card transactions up to 54 percent, thanks to algorithms based on machine learning.
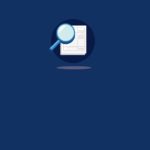
For years, banks have been using machine learning techniques to detect card fraud. Algorithms are trained to learn to recognize behavioral patterns typical of fraudulent transactions and block subsequent 'suspicious’ operations.
The drawback? The false positives. When customers pay an unusual amount of money or use their cards far from their usual locations, their cards often get blocked because the system erroneously qualifies these anomalies as “suspicious”.
The reason for this is that these techniques – quite successful in other fields – are subject to a series of limitations when applied to card fraud. As their ability to detect actual fraudulent transactions grows, so does the number of false positives they derive; in other words, the number of legitimate transactions they erroneously label as fraudulent.
The obstacles
One of BBVA’s permanent challenges is to improve user experience in these situations without compromising the current security levels. With this goal in mind, BBVA’s Corporate Security and Engineering Risk (CS&ER) team began collaborating with MIT’s Computer Science and Artificial Intelligence Lab (CSAIL) in 2015 to try to find new ways of reducing false positive levels. The outcome of this collaboration was published as a joint study, which MIT recently presented at the European Conference for Machine Learning. It was also included in an article on MIT’s news portal.
One of the problems identified based on MIT’s work was the scarcity of mathematical variables (known as “features”) that could be inferred from the data used to train machine learning systems. In other words, the limited amount of detailed information available to train the algorithms about actually suspicious behaviors.
Another problem is the scarcity of useful examples to train models, what researchers equate to “looking for needles in a haystack.” Algorithms must be capable of identifying anomalies that happen on very rare occasions. In Spain, only 17 out of every 100,000 card transactions are fraudulent, according to the Bank of Spain.
The solution
The MIT and BBVA research team applied groundbreaking automated feature engineering techniques to try to improve this situation. They used the Deep Feature Synthesis method, a model developed by two MIT researchers, Kalyan Veeramachaneni and James Max Kanter, which makes it possible to derive highly detailed features from datasets in an automated manner.
This new approach allowed the research team to derive over 200 new additional features from every transaction. These features can be used to describe the behavior of card transactions in greater detail and improve the results yielded by the detection engines. Some of these new features used to fine tune true positive results indicate, for example, whether the user was physically present while the transaction was taking place, or the average time spent in certain stores. This helps to more accurately identify when a particular card user’s transaction veers from the norm.
So far, tests are yielding promising results. False positives have dropped dramatically, while keeping the same level of fraud detection, compared to other existing products and systems. Specifically, applying the model to a dataset of 1.8 million BBVA transactions cut the number of false positives by 54% over traditional models, saving the bank approximately €190,000.
"Taking as a starting point this model that was designed and prepared by the MIT team, as internal research in the bank, we have synthesized new features using additional card and business data that were not available at the time of the original research,” explains Carlos Capmany, who manages the project for BBVA’s CS&ER area.
“As a result, we have managed to consistently reproduce the already impressive results of false positive reduction obtained by the MIT research team, and this with a minimum increase in the overall computational cost of the system," adds Capmany.
The benefits of the model
Applying the results of this research to BBVA’s fraud detection systems has proven that they have the potential to improve customer satisfaction in card transactions without compromising current security standards.
Also, BBVA’s CS&ER area is using this technique developed by MIT in other fields of research on machine learning applied to cybersecurity with similar constraints: few variables to train the machine learning system and disparate input data.
“This automated feature synthesis technique and the overall knowledge MIT contributed to this project have shown us a new way of refocusing research in other challenges in which we initially have a reduced set of features. For example, we are obtaining equally promising results in the detection of anomalous behavior in internal network traffic or in market operations, just to mention two," adds Sergio Iglesias, another member of the CS&ER team, who participated in the project.