'Machine learning': intelligence that learns by itself
Below a mountain of 'big data' lie simple laws that allow you to define patterns. 'Machine learning’ uses them to improve the lives of human beings.
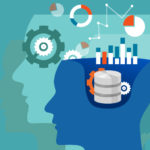
Machine learning – automated learning - allows machines to learn without being expressly programmed. This learning ability is essential to develop smart systems, capable of identifying patterns and turning data into forecasts.
"When you are programming, you tell the data what the next step is. With machine learning that control is reversed: it's the data that tell you what the next step will be," explains Keepcoding co-founder Fernando Rodríguez, speaking at 'Big Data & Machine Learning. Millions of datum, Endless Possibilities’, an event held at BBVA's innovation Center.
The term ‘machine learning’ is used to refer to the ability of systems to generate their own algorithms based on the data and results we want to find out about. "Underneath a mountain of data lies a simple law that explains behaviors and allows defining a pattern", explained the expert.
Cristóbal Sepúlveda, Technical Architect at BBVA, said at the event that this branch of artificial intelligence (AI), machine learning, "allows the bank to solve problems without having to re-program its systems." These systems created with the help of machine learning not only provide answers, but are also learning to ask smart questions to turn data into forecasts and formulate their own hypotheses.
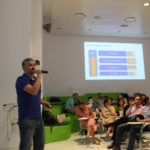
Cristóbal Sepúlveda, Technical Architect at BBVA, during the event.
According to the BBVA expert, AI also allows the bank to get to know its customers much better, and thus deliver increasingly “better services and experiences.” "It also helps us as employees, because by having much more powerful tools, we are now able to create much more innovative solutions," he said.
The years of trial and error
One of the defining aspects of machine learning is that it requires tremendous amounts of both data and processing power. And that is something that was just not available in the 1980s, as Keepcoding expert Rodríguez recalls: "In addition to shoulder pads and the A-Team, artificial intelligence caused quite a stir in that decade, but the hype faded away nobody was able to deliver on the unrealistic promises that were thrown around.”
Indeed, many experts refer to the 80’s as the "AI Winter". During these years, said Rodriguez, three factors led to the demise of machine learning: First, the approach to the problem was inappropriate: people tried to use highly advanced algorithms to solve very simple problems. Second, the overall lack of processing power meant that computers were just not able to implement AI algorithms. And third, the lack of data availability and storage capacity.
But all this changed with the advent of the internet: "Data generation, in terms of volume, speed and variety, exploded." This led to an "avalanche of data" that, as noted by Rodríguez, is being used, in the case of banks, to assess risks or detect fraudulent use of credit cards, for example. "Now you can detect patterns and predict future behaviors, something that requires having tons of data," he said.
Big data based recommendations
Sepúlveda also exposed an actual use case of this technology: "At BBVA, we developed a service recommendation engine for bank users," With this proposal, what we are trying to do is offer the best commercial offer depending on the most used transactions by the user and their navigation patterns. All this information is processed in a classification algorithm which then generates a recommendation. "The volume of information is incredibly vast and the only way to offer a recommendation is using machine learning technologies", he noted.
Although the benefits are great, there are also risks to the use of machine learning. One of them is "overtraining,” which causes systems to exclusively detect the patterns they encounter during training phases. Rodriguez explained the problem through an anecdote: Allegedly, the US Army once developed a tank detection software that worked flawlessly in the laboratory. "However, when they tried take it to the real world, they realized that something was failing: it was unable to detect tanks," explains the expert. The problem was that he had been trained with images of tanks under cloudy skies. That turned the software into "a fabulous cloud detector" when used outdoors.
*If you are interested in Big Data & Machine Learning, connect with BBVA at LinkedIn to get related information and info on similar topics