Superheroes fight AI algorithms' gender bias
Many organizations now process huge amounts of data using artificial intelligence. So it is crucial to find ways to prevent unfair biases. New research by BBVA and the Universidad de Barcelona (UB) tries to achieve this with a model that automatically sorts more than 600 superheroes as “good” or “evil” while disregarding traits like gender or race.
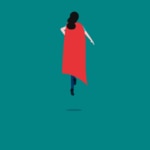
Irene Unceta, a data scientist at BBVA’s Artificial Intelligence Factory, with co-authors Jordi Nin (now a professor at ESADE) and Oriol Pujol, of the Universidad de Barcelona, have published research showing that machine learning models that skip sensitive data, like gender or race, can still be good at decision-making.
“Our goal is to prove that bias can be mitigated in data-driven predictive models without watering down their effectiveness. This means algorithms don’t need to make decisions based on sensitive data,” said Irene Unceta, one of the authors of the paper made public at the ninth Iberian Conference on Pattern Recognition and Image Analysis. The researchers’ clever idea was to train a specially designed sorting model on a database of 600 fictional superheroes that fully describes their physical traits, personal qualities and superpowers.
“The fact that a superhero is male or female, a mutant, a robot or a human should be irrelevant to whether they are good or evil, so the model should train without using that kind of information,” Unceta explained. The research is part of the industrial doctoral program underway at the Department of Mathematics and Computer Science of the UB, in partnership with BBVA AI Factory.
The first step was to develop a model that looks at all available information, like skill levels across a hundred superpowers, hair color, height and weight—but also potentially sensitive data about each superhero’s race or gender. Next, the research team copied and pasted the decision-making structure this model had learned into a new model that is race- and gender-blind.
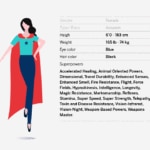
Unceta, Nin and Pujol found that the two models had similar predictive power. And the second model almost entirely eliminated differences in predictive power between women and men because it disregards gender. While the original model tended to be worse at sorting males than females, the second model’s predictive power was balanced across all the fictional characters regardless of gender.
Specifically, the original system was 9% worse at classifying male superheroes than it was for female superheroes. But the race- and gender-blind model narrowed the difference to just three points.
The paper also highlights that the gender-blind model, even though it has less room to evolve because it copies over the original model’s decision-making structure, makes up for the missing information by tweaking the final decision-making stage, thus correcting for learned biases.
“The fact that a superhero is male or female, a mutant or a human, should be irrelevant to whether they are good or evil"
“The second model tries to fill in missing information with small changes, which it makes automatically. Since it is constrained to replicating the behavior of the first model, its freedom of movement is tightly limited, so it’s forced to make small concessions. Yet it is precisely those concessions that mitigate the bias ingrained in the original model,” Unceta said
What makes this research so exciting is that it suggests a method that could mitigate machine learning biases by removing the need to train the algorithm using sensitive data. This approach might not always work, however. The paper specifically warns that the information could “leak out.” Leakage happens when data points are correlated, so even if the sensitive data that might trigger discrimination were eliminated, you could still correctly guess what it was by looking at the remaining data.
Despite that drawback, the method opens the door to creating fairer models. This approach could be used in the real world to detect and remove biases in sorting models, and to check that algorithms make decisions fairly.
Moreover, the authors of the paper think that fairness checks on data-driven sorting models could become more sophisticated in future.
This research effort is a first step toward developing algorithmic models that are unbiased but just as accurate. Removing bias is one of the main ethical challenges faced by artificial intelligence, and a hot topic of debate in academic, government and business circles: we need to make sure that the biases or prejudices embedded in the data itself won’t taint AI-driven decisions.