As we have seen in previous articles, the intelligent use of data, with the right talent and the pertinent business strategies for applying creative solutions, can provide insights that help solve a whole gamut of problems and take on board new opportunities. Data places within our grasp an enhanced decision-making ability and a deeper understanding of our clients and what they need. But as in the case of any other tool, these new skills can be put to positive or not so positive use, whether intentionally or not.
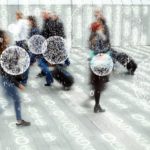
Let's take a look at the risks involved in the use of data and algorithms from the point of view of society's trust in new digital applications, in the following basic areas: self-regulation and transparency, data literacy, which is precisely the objective of this article, and finally the design of people-oriented services, using data to resolve human problems.
Types of data
To better understand the implications of the use of data, we need to enhance our awareness of how they are categorized. The first distinction we must make is between “object-related” data and “person-related” data.
An example of the former is the cartography of a city, the representation of its streets, parks, buildings, public and private services and all of the changes these undergo through time. This data can be recorded and organized by the public sector (National Geographic Institute, regional cartography services), or by private companies (Google, Apple). The use of such sources is regulated by the terms of use defined by the institution that gathers the information, processes it and makes it available to those who want to use it.
However, use of the latter category of data also requires the express permission (consent) of the person referred to in the information, in the same way that a photographer cannot make free use of an image of a person. Although the camera and memory card in which the image is stored may be his property, the use of a personal image requires consent, as it could affect the individual's right to privacy. This is the case of data gathered and processed by private companies as a service to their clients. It can be classified in the following way:
A) Data provided directly by the user (e.g. the case of a home address provided when contracting a service)
B) Directly-observed data (e.g. data recorded by a bank on card payments that have been authorized)
C) Inferred data (e.g. predisposition to contract certain services, obtained as the output of a model that uses input of declared or observed data or from sources of type D)
D) Data gathered by third parties.
It’s important to point out that in any of these cases, it is necessary to obtain the express agreement of the person before the data is used, as stipulated by the European Union’s General Data Protection Regulation (GDPR).
Years ago, at the start of the digitization of information systems for a company (or a government), clients (or citizens) were classified by a very limited range of vectors, basically type A. Nowadays, people are defined by a far more extensive range of variables. Types B and C have increased both in numbers and importance, and in some cases combined with D types, if the client has provided the necessary authorization. The increase in the depth of information available, along with the ability to make connections between a multitude of facts that were previously undetected will help form decisions that affect people. This places a heavy burden of responsibility on those of us who work such data.
Even with support from augmented intelligences, the people that rely on them are still responsible for the actions"
Risks in the development of data-based solutions and how to avoid them
Misleading information regarding, on the one hand, which data a company collects and for what purposes, together with, on the other, privacy violations. The appropriate protection of personal information, guaranteeing its security and the implementation of information channels that allow to easily exercise the rights of access, rectification, opposition or deletion, together with a strict interpretation of the legal and ethical framework, are pivotal in avoiding these risks. One of the most severe risks is the unauthorized use of data (either data disclosed by the person, or inadvertently collected.) Any new data-based application proposal has to be based on transparency and self-regulation.
- Dysfunctional solutions: An excess of reliance on data and algorithms, or pressure to launch applications before they can be properly validated, can lead to inaccurate responses. Errors in the cartographic information offered by some navigation systems have caused accidents and we all can imagine the implications of a false negative in a clinical diagnosis. Solving these issues requires a two-pronged approach: First, sound data governance should ensure data quality and, second, algorithmic audit and peer review processes should guarantee the methodological rigor and validity of the solutions before they are released.
- Unfair bias: Since we’ve gone from programming machines to helping them think, an analytical model can reflect biases implicit in the data used in the learning process and which may be discriminatory against certain minorities. It is our duty to be aware, control and mitigate the biases existing in the training data, and refrain from using artificial intelligence to widen preexisting divides. Also, we cannot excuse ourselves behind the inscrutability of the models that neural networks use. When applying them to business decisions, the variables that weighed the most in the final decision need to be identified in so far as possible, to inform the affected individuals, in a transparency exercise aimed at helping them correct everything they can on future occasions. Even with support from augmented intelligences, the people that rely on them are still responsible for the actions.
It is our duty to be aware of the biases existing in the data and refrain from using artificial intelligence to widen preexisting divides"
The keys to using data responsibly
To conclude this reflection, we recommend reading the article, entitled “Ten simple rules for responsible big data research.” We were very pleased to confirm that everything we do at BBVA Data & Analytics abides by these rules.
- Internally: We realize that the data we work with refer to people, and we apply security standards to adequately protect the information (rules 1 & 2). In our pursuit of maximum rigor in terms of results, we’ve established the peer-review mechanisms that facilitate the algorithmic audit thereof (rules 7 and 8), and we also engage in active discussions regarding the implications of the models we develop (rule 6), always keeping a healthily critical point of view whenever excessive expectations build up in relation to data whose quality or biases have not been properly measured (rule 5), but we also try to help our colleagues from other areas realize what opportunities innovation offers beyond rigid frameworks, in those grey areas that remain unexplored, where the only guide are our self-regulation criteria (rule 10).
- Externally: When we want to share data, for example, with academic research teams, we anonymize information by applying the pertinent standards (rule 2 and 3). But this openness has not been limited to the field of research; we believe that data provide the soil that will nurture innovation, we’ve created open anonymized statistics sharing tools to support the development of new business models and also data applications that seek to contribute to the greater good (rule 4). In this same sense, we’ve expanded our focus to encompass society as a whole through analyses that go beyond the scope of our core business (rule 9).
We want to go one step further: we want to share the information we’ve gathered about our customers with them, organizing it in a way that’s most useful for them. We want to develop solutions based on customization, convenience, immediacy and tailored and useful advice, building on data and algorithms. And we want to do this while preserving our most valuable asset: our customers’ trust
*This is the third article in a series exploring the challenges and opportunities of Data in the digital world. Read the first one here and second one here.