Artificial intelligence, the financial sector’s ally to curb risk
With investments on artificial intelligence expected to reach €10 billion by 2020, it is evident that the financial sector is very optimistic about these technologies. However, the level of uncertainty surrounding AI remains almost too high to justify this degree of enthusiasm. In a recently published paper, the World Economic Forum warns about how overhyped misconceptions of what AI really is and what it can deliver may undermine the potential benefits derived from the use of artificial intelligence in finance. In contrast with this vision, other experts concur that AI contributes more to prevent and mitigate risks than to generate them.
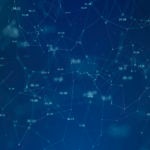
Data is a key component of risk management activities across all sectors of economic activity, including the financial industry. Sometimes, analyzing the sheer volume of data being generated today to detect risks becomes a daunting task. At the same time, managing data adequately is becoming increasingly pivotal for companies striving to prevent cases like Facebook’s. That is why many institutions are leveraging the latest technological advances to enhance their risk detection processes, deploying artificial intelligence capabilities.
Thus, the use of artificial intelligence and machine learning to manage risks is especially convenient when handling and analyzing unstructured data sets – i.e. data that cannot be easily classified into categories. Cognitive technologies, such as natural language processing tools use advanced algorithms to analyze text in order to derive insights and sentiment from unstructured data, according to a report by Deloitte.
According to this same report, taking into account that 90 percent of the data generated today is unstructured, implementing cognitive analytics can put businesses right on the cutting edge. Leaders who leverage cognitive technologies to anticipate and proactively manage risk can gain competitive advantage and use risk to power their organizations’ performance.
Another article published by Ernst&Young emphasizes the advantages of creating sophisticated tools to monitor and analyze behavior and activities in real time. As the article explains, since these systems can adapt to changing risk environments, they can evolve from early warning systems into early learning systems that prevent threats materializing for real.
Real-life examples of the use of AI and machine learning
There are several real-life examples of how AI can be used to prevent and mitigate risks. One would be fraud detection. Thanks to AI, it is possible to not only to analyze higher amounts of data to detect fraudulent activities, but also learn and generate standardized patterns that save a lot of effort when detecting a similar fraud again. In other words, if cognitive analysis identifies something as a fraud, the machine learns and the next time it will be able to automatically detect it. The computer learns and becomes more and more intelligent. And as cognitive fraud detection systems learn, they will be able to detect more complex frauds, an advantage that has a great impact in terms of risk management.
Another example is the use of machine learning to back more informed forecasts about how likely individuals or organizations are to meet their loan or payment obligations, or to build more accurate income forecasting models. Thus, the capacity of these automatic learning models to analyze massive datasets —financial and non-financial— can help boost the analytical capabilities in risk management and regulatory compliance, helping analysts make more informed decisions.
These systems can also help detect fraudulent behaviors within organizations. Automated systems can help financial institutions monitor their traders’ activities by matching their commercial information against other sources of information, including email traffic or their calendars. The applications are multiple: Monitoring, risks generated by suppliers, detecting cyber attacks, etc.